Starting Date: June 2024
Prerequisites: linear algebra, calculus, general machine learning and data analysis concepts, python
Will results be assigned to University: No
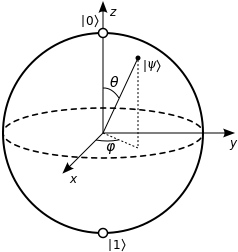
Quantum Technologies (QT) may revolutionise data science but are often unreliable. Classical and quantum noise makes most of the existing systems highly unstable. This generalised unreliability has limited their applicability to real-world computational problems.
In special cases, quantum systems can be simulated on classical computers. As classical simulations are noise-free, they can be used to understand whether they produce sensible or untrustable outputs.
In this project, you will train a Neural Network (NN) to predict the noise-free output of quantum circuits. The model inputs are the features of the circuit and the initial quantum state. After training the network, you will try to predict the output of circuits that we can not simulate classically. See [1] for a recent implementation of this idea.
[1] Liao, Haoran, et al. “Machine learning for practical quantum error mitigation.” arXiv preprint arXiv:2309.17368 (2023).